Bayes' Theorem for Beginners
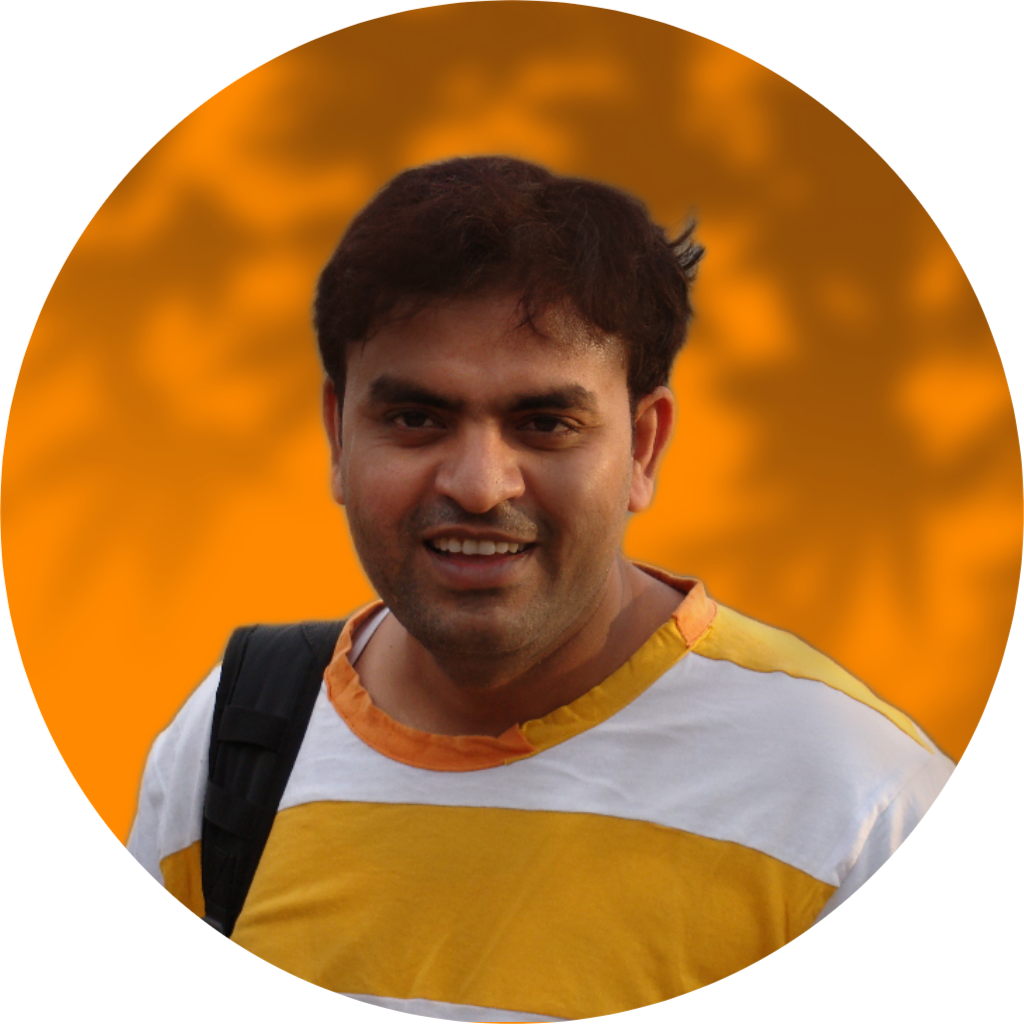
webadmin
Shared publicly - September 23, 2024
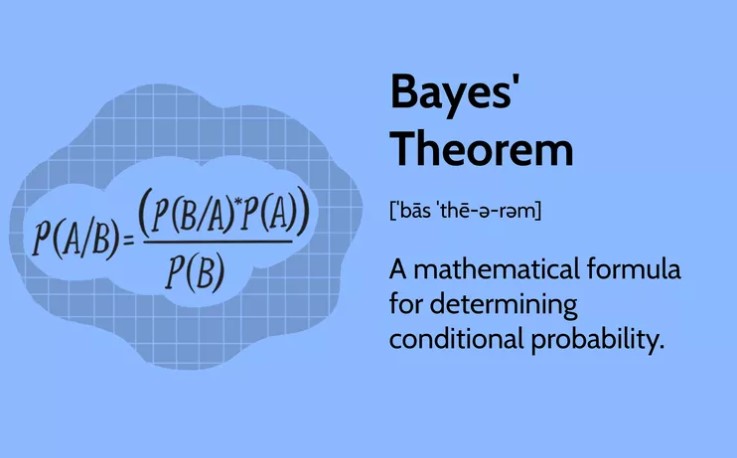
Bayes' Theorem for Beginners
Bayes' Theorem is a key concept in probability theory. It helps us update the probability of an event
based on new evidence or information. This is especially useful in many fields like artificial
intelligence, statistics, and everyday decision-making.
What is Bayes' Theorem?
Bayes' Theorem allows you to calculate the probability of an event occurring given that another
event has already happened.
The formula is:
P(A | B) = [P(B | A) * P(A)] / P(B)
Where:
- P(A | B) is the probability of event A happening given that B is true (this is what we're looking for).
- P(B | A) is the probability of B happening given A is true.
- P(A) is the probability of A happening (before we know B).
- P(B) is the probability of B happening.
Simple Example of Bayes' Theorem
Imagine you are a doctor, and a patient tests positive for a rare disease. The test is 99% accurate, but only 1 in 1,000 people actually have the disease.
We want to know: What is the probability that the patient actually has the disease, given the positive test result?
- Let's break this down using Bayes' Theorem:
- P(A) = Probability that the patient has the disease = 1/1000 = 0.001.
- P(B | A) = Probability of a positive test result given the patient has the disease = 0.99.
- P(B) = Overall probability of testing positive, including false positives and true positives.
Using the formula, we can calculate the true probability of having the disease even after a positive test result.
Why is Bayes' Theorem Important?
Bayes' Theorem is important because it provides a way to think probabilistically. It is used in many areas, including:
- Medical diagnostics
- Spam filters
- AI and machine learning
It allows us to update our predictions or decisions based on new evidence, improving accuracy in uncertain situations.